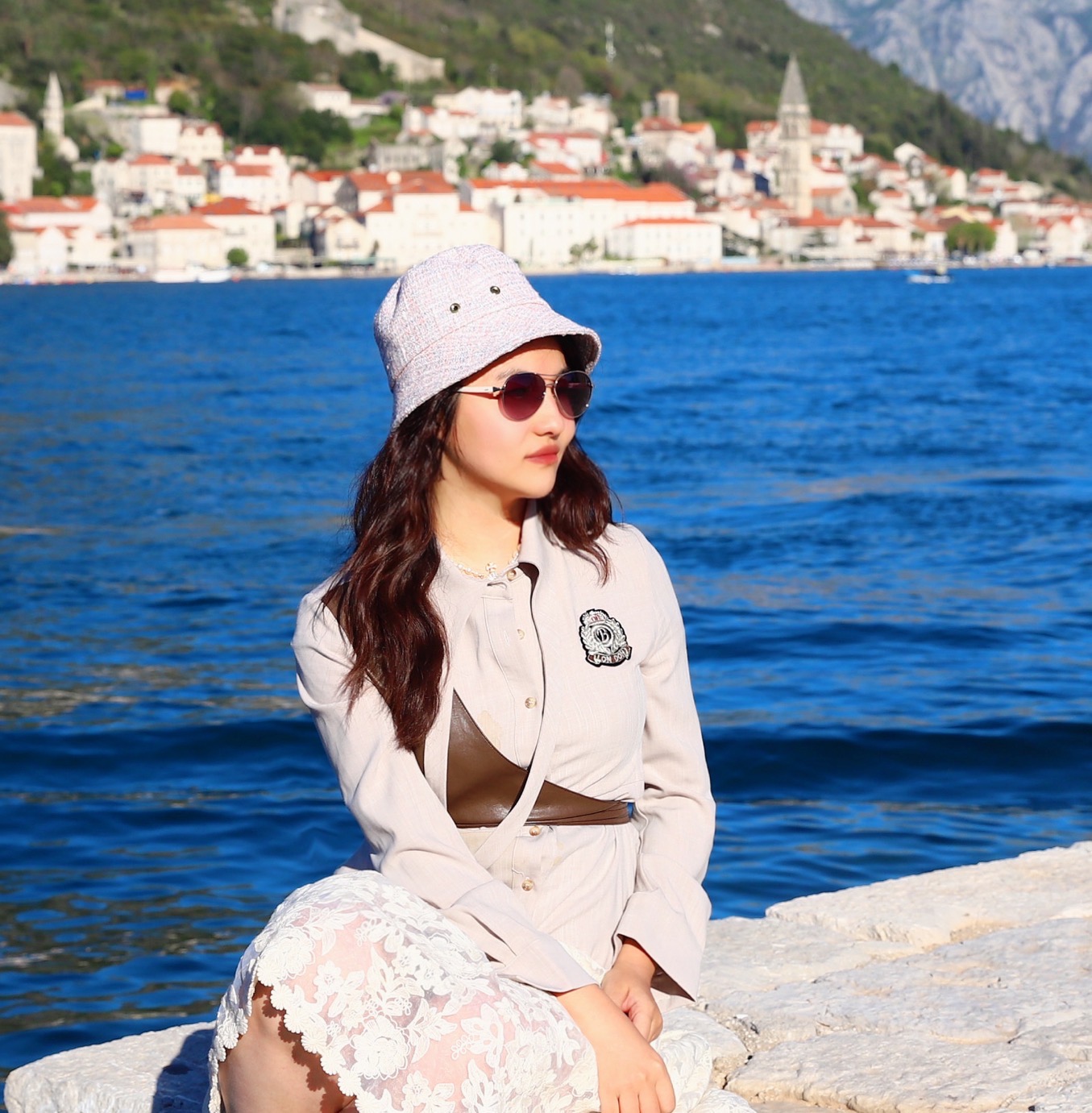
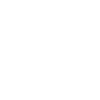
In the sweltering heat of Shanghai, Jazzyear had the privilege of meeting Professor Jürgen Schmidhuber, a distinguished guest at the 2024 World Artificial Intelligence Conference (WAIC).
Based on years of earlier research, Schmidhuber and his student Sepp Hochreiter published the architecture and training algorithms for Long Short-Term Memory (LSTM) networks in 1997 in a journal. This type of RNN (Recurrent Neural Network) is widely used by tech giants for applications in natural language processing, speech recognition, video games, and more, including Apple’s Siri and Google’s translation services. Prior to the advent of ChatGPT, LSTM was heralded as “the most commercially valuable achievement in AI.” The 1997 LSTM paper is the most cited AI paper of the 20th century, perhaps even the most cited computer science paper of the century.
Even earlier, in his “Annus Mirabilis” 1990-1991, Schmidhuber laid the foundations for Generative AI, by introducing the principles of what ‘s now called GANs (Generative Adversarial Networks), non-normalized linear Transformers, and self-supervised Pretraining. These 3 contributions correspond to the “G,” “P,” and “T” in ChatGPT, where GPT stands for Generative Pre-Trained Transformer.
Long before the so-called “Deep Learning Trio” shared a Turing Award, Schmidhuber was already being hailed as the “Father of Mature AI” by The New York Times. Elon Musk also praised him on X, stating, “Schmidhuber invented it all.”
In 2013, the International Neural Network Society (INNS) awarded Schmidhuber the Helmholtz Award. In 2016, he received the IEEE Neural Network Pioneer Award in recognition of his "pioneering contributions to deep learning and neural networks." Currently, he serves as Scientific Director at the Swiss AI Lab IDSIA and heads the AI initiative at King Abdullah University of Science and Technology (KAUST) in Saudi Arabia. He is also involved with several AI companies.
Therefore, this 61-year-old German luminary has sparked a discourse: Why has Schmidhuber not won a Turing Award?
This is a key concern for many industry professionals, but it does not include Schmidhuber himself. During our two-day in-depth conversation, Schmidhuber, donning his signature stylish black beret and speaking fluent English with a German accent, came across as a scholar who combines humor with approachability. Yet beneath this amiable exterior lies an indomitable spirit, eager to establish scientific integrity in the fast moving field of AI research.
Discussing overlooked contributions of AI pioneers—particularly groundbreaking advancements achieved by small European labs before the tech giants took notice—Schmidhuber expressed a palpable urgency to correct misleading historical records of AI.
For years, he has engaged in public debates with Yann LeCun, Geoffrey Hinton, Yoshua Bengio, Ian Goodfellow, and others, accusing them of rehashing not only his own earlier work, but also the earlier work of others, without citing the original inventors. He backs up his claims with numerous peer-reviewed references.
Schmidhuber’s forthrightness naturally stirs controversy. However, his perspective provides a valuable counter to a widespread yet misleading Silicon Valley narrative. Moreover, he not only speaks up for himself and his outstanding students, but also tirelessly champions other underappreciated contributors to the AI field, striving to give them their due recognition.
Regarding the debate over who should be called the “Father of AI,” Schmidhuber points out that one needs an entire civilization to build an AI. He also points out that modern AI is driven by mathematical and algorithmic principles discovered decades and even centuries before the term “AI” was coined in the 1950s.
As for the controversy online, Schmidhuber remains unfazed. He quotes Elvis Presley, saying, “Truth is like the sun. You can shut it out for a time, but it ain’t going away.”
In this exclusive interview with Jazzyear, Schmidhuber discussed the origins of AI, which greatly predate 1956, his own research that laid the foundations of modern AI, his view of the “Deep Learning Trio,” as well as the cosmos-changing future of self-replicating, self-improving machine civilizations. He also believes that, on the journey toward AGI, even those without significant funding can bring about revolutionary changes in AI research.
1. Something better than the Transformer
Jazzyear: Let’s start with the history of artificial intelligence. You have a deep understanding of AI’s development. What do you think needs to be clarified about AI’s history?
Schmidhuber: Oh, there is a lot. For example, the origins of AI date back well before the 1956 Dartmouth Conference, which is sometimes cited as AI’s inception point, because there the name “AI” was coined for a field that was already old by then. In fact, as early as 1914, the Spaniard Leonardo Torres y Quevedo designed a chess-playing automaton at a time when chess was considered a domain exclusive to intelligent beings. The theory of AI can be traced back to Kurt Gödel’s work in 1931-1934 when he identified fundamental limitations of what can be computed by any AI.
Some claim that artificial neural networks (NNs, now widely used) are a relatively novel concept from the 1940s and 50s. However, “modern” neural nets date back over 200 years ago. Around 1800, Carl Friedrich Gauss and Adrien-Marie Legendre introduced what we now call a linear neural network, though they called it the “least squares method.” They had training data consisting of inputs and desired outputs, and minimized training set errors through adjusting weights, to generalize on unseen test data: linear neural nets!
This was what’s now called “shallow learning.” Some think that the more powerful, more recent “deep learning” is a 21st-century innovation. It isn’t. In 1965, in Ukraine, Alexey Ivakhnenko and Valentin Lapa had the first working deep multilayer networks that learned. For example, Ivakhnenko’s 1970 paper detailed an eight-layer deep learning network. Regrettably, certain much later publications on very similar approaches failed to credit the Ukrainian pioneers. Our field is rife with such cases of inadvertent or deliberate plagiarism.
Jazzyear: You have also played a pivotal role in AI history. Could you talk about the miraculous year of 1991? What contributions did your research make to the AI industry during that period?
Schmidhuber: Our miracle year of 1990-91. That's something I'm really proud of. At the Technical University of Munich, we were lucky enough to publish many of the basic concepts behind today's AI, in particular, Generative AI.
The GPT in ChatGPT stands for Generative Pre-trained Transformer. Let’s first look at the G in GPT and in “Generative AI.” Back in 1990, I had what’s now called a Generative Adversarial Network (GAN), which I initially dubbed “Artificial Curiosity.” This involves two competing neural networks—a generator with adaptive probabilistic units and a predictor influenced by the generator’s output. The predictor tries to predict how the environment will react to the outputs of the generator. It minimizes its loss by gradient descent. However, in a minimax game, the generator tries to maximize what the predictor is minimizing. Essentially, it aims to “fool” the adversary by generating surprising content. This idea later found extensive use in Deepfake applications.
As for the “P” in GPT, it refers to Pre-training, another concept I published in 1991. I saw that unsupervised or self-supervised Pre-training can greatly compress sequences, facilitating downstream deep learning for long sequences, such as very long texts.
Then there’s the T, which stands for a neural net called Transformer. The name “Transformer” was coined at Google in 2017. However, I had already introduced variants of the concept in 1991 under the term “fast weight controllers” or “fast weight programmers.” One of my variants is now called the “unnormalized linear Transformer.” It was even more efficient than the modern Transformer, because it scaled linearly, requiring only a hundredfold increase in computational power for a hundredfold increase in input size, unlike today’s “quadratic” Transformers, which need a ten-thousandfold increase.
Jazzyear:Many, including the creators of the quadratic Transformer, have stated that we need something better than it. It is certainly not perfect. What do you think the next generation of architectures should look like?
Schmidhuber: The 1991 linear Transformer above is actually a good starting point for making quadratic Transformers more efficient. However, to predict the next generation of large language models (LLMs), let’s first revisit the first generation. The first LLMs of Google and Facebook utilized our Long Short-Term Memory (LSTM) recurrent neural network (RNN), which also has roots in 1991, namely, in the thesis of my brilliant student Sepp Hochreiter. This thesis not only described experiments with the aforementioned pre-training (the P in ChatGPT), but also introduced residual connections, essential for deep learning and handling long sequences. I coined the term LSTM in 1995, but the name is not important, the only thing that counts is the math. LSTM was used for LLMs until the late 2010s when Transformers, easier to parallelize and thus advantageous for modern hardware like NVIDIA GPUs, took over.
Jazzyear:Can RNNs solve tasks that elude Transformers?
Schmidhuber: Yes, they are more powerful in principle. For example, parity: consider bit strings such as 01100 or 101 or 1000010101110. Given such a bit string, is the number of 1s odd or even? Looks like a simple task, but Transformers fail to generalise on it. However, even simple RNNs can quickly solve this task, as I showed decades ago.
Recently, Hochreiter’s team developed an impressive LSTM extension called xLSTM, with linear scalability, outperforming Transformers on various language benchmarks. Its exceptional understanding of text semantics, alongside its versions that can be highly parallelized, makes xLSTM a compelling candidate for future large-scale experiments.
2. What is the linear way of thinking?
Jazzyear: You are now leading the AI initiative at King Abdullah University of Science and Technology (KAUST) in Saudi Arabia. What drew you to take up this position?
Schmidhuber: Before that, I mostly worked in Switzerland, which is a great place for science, leading the world in terms of Nobel prizes, patents, citations, and AI publications per capita. But they don’t have a place like KAUST, which is now the university with the highest impact per faculty, surpassing institutions like Caltech and Princeton. KAUST seemed like a great opportunity to push AI research further. Saudi funding benefits the rest of the world, since we are producing lots of open-source results that are accessible from China, the USA, and other places.
Jazzyear: I’m sure KAUST has substantial funds and resources for AI research. Is this why big companies are more attractive? Have small independent teams or academia become unable to achieve significant breakthroughs?
Schmidhuber: What constitutes a “significant breakthrough”? Is it the next foreseeable 0.5% performance boost of LLMs, demanding lots of computational power and money? Or is it a real breakthrough that makes the next AI as energy-efficient as a human brain? I still believe that someone with a good idea can revolutionize AI research without requiring enormous resources.
Jazzyear: Based on your diverse experiences, how do you view the differences in AI academia and industry across the US, Europe, the Middle East, and China?
Schmidhuber: Europe, of course, is the origin of computers, computer science, AI, deep learning etc. Most of modern AI originated there. In particular, almost all of the core deep learning techniques were developed in the previous millennium in Europe (although there were also important contributions from Japan). Europe is still producing a lot of AI talent. At some point, however, scaling became crucial, and that’s where the US and China took over. Europe lacks tech giants like Google, Apple, Facebook, Amazon, Alibaba, TenCent, etc, although all these companies are based on the WWW, which also originated in Europe around 1990. Today, the US and China have numerous unicorn startups, whereas Europe has just a few. Large US companies with huge market valuations can easily buy some of Europe’s best talents and entire startups such as DeepMind.
Jazzyear: How do you think DeepMind has developed recently? Earlier achievements like AlphaGo and AlphaZero were published in Nature or other journals. But now, the trend is more towards OpenAI’s model of delivering excellent products directly to users.(Shane Legg, co-founder of DeepMind, was a PhD student in Schmidhuber’s Swiss lab)
Schmidhuber: DeepMind once was almost like academia without its drawbacks. Their well-funded researchers could publish without having to worry about writing grant proposals or teaching. However, even a star company like DeepMind was unable to sustain independent growth. It was sold to a much bigger US company even before it became a unicorn valued at $1 billion. Now it’s just a small part of Google.
Jazzyear: There’s always a comparison between academic labs and big companies. Aditya Ramesh, one of the heads of OpenAI’s video generation product Sora, recently said that academia can mainly do evaluation and measurement or research AI explainability now, lacking resources like GPUs to make more significant contributions. By the way, Aditya doesn’t have a PhD because he joined OpenAI right after his undergraduate studies.
Schmidhuber: Did he really say that? Anyway, such statements seem a bit naïve, reflecting a very linear way of thinking: the current trend is to scale large foundation models through more and more compute, and since some people cannot imagine anything else, let’s just extrapolate the current trend, everything else must be useless!
Jazzyear: So, you are definitely not a fan of scaling law?
Schmidhuber: I am the biggest fan of the old scaling law that says: every 5 years compute is getting 10 times cheaper (this law has held since 1941 when Konrad Zuse completed the first working general purpose computer in Berlin). That scaling law is the reason why our techniques from the 1990s are now on billions of smartphones. AI is getting 100 times cheaper per decade, and everybody will profit from this, not just a few big companies.
It’s just that the scaling of present LLMs has little to do with AGI (Artificial General Intelligence) that learns like humans learn. How does a baby learn? Not by downloading the web. It learns to collect data through self-invented experiments that improve its adaptive neural world model, which it can use for planning. All of this, however, has little to do with the LLMs that are now so popular.
Corporations must maximize shareholder value, whereas scientific research seeks unprecedented discoveries. Don’t expect these quite different objectives to be aligned!
Jazzyear: But money issues are always crucial, even for science.
Schmidhuber: Sure, for centuries, science and art have followed the money. For example, in the 1980s and 1990s, well-funded labs in rich countries such as Japan and West Germany were the cradle of innovations such as CNNs and Generative AI, respectively. Around 1995, the combined nominal GDP of these two tiny countries, the 2 big losers of World War II, exceeded the GDP of the US (the Chinese economy was still small back then). Today, only 3 decades later, the US and China are much bigger economically (since 2017, China has been number 1 in terms of PPP), and the scaling up of these old inventions mostly happened on the Pacific Rim in industrial labs.
I recall when I first came to China 15 years ago, I had to show taxi drivers a picture of my hotel to tell them where I was going. Now, they just lift their smartphones, and I speak the destination in English or German, and they understand. My taxi driver may not know that this is based on research results from my lab, but it’s those resourceful and powerful companies that have popularized these technologies, helping to profoundly transform daily life. They are the ones who took academic inventions and made them accessible services.
3. They should be stripped of their awards
Jazzyear: Do you like being called the “Father of Modern AI”?
Schmidhuber: A single person cannot create an AI from scratch. You need an entire civilization to build an AI. You need people to create the basic algorithms, others to build computers, others to mine the materials from which the computers are made. You also need consumers, like gamers, who drive the demand for faster computers, and farmers who grow the food for all.
Jazzyear: That’s interesting. Everyone plays a role in the creation of AI.
Schmidhuber: Of course, you can trace specific neural networks to their creators. For instance, the father of convolutional neural networks (CNN) is Kunihiko Fukushima, who published the basic CNN architecture in 1979 in Japan. In 1987, Alex Waibel, a German working in Japan, combined convolutions and backpropagation, the method published 1970 in Finland by Seppo Linnainmaa, now widely used to train neural nets. Zhang (1988) published the first backprop-trained two-dimensional CNNs, also in Japan. Thus, from 1979 to 1988, modern CNNs as we know them originated in Japan.
Jazzyear: You have yet to receive the Turing Award. Is this a significant regret, or do you not concern yourself with it?
Schmidhuber: How much should you care about an award that was also given to people who republished key methods and ideas whose creators they failed to credit? is a total no-go in science, please check my report on this on the web.
Jazzyear: Apparently you are referring to the European-born Deep Learning Trio—Geoffrey Hinton, Yoshua Bengio, and Yann LeCun—who won the Turing Award. You mentioned they have not adequately cited or acknowledged your earlier work. Has your opinion of them changed?
Schmidhuber: No. They frequently re-published others’ work without proper attribution. Even worse, they failed to correct this in subsequent publications. In science, this is unacceptable. My team has particularly suffered from this behavior; their most prominent work directly builds on ours. But this isn’t just about my own team; relevant work of many other scientists has been republished by them without proper attribution.
Jazzyear: Do you think they owe you an apology?
Schmidhuber: They have never apologized in the past when they had a chance, and have never corrected their papers accordingly. They have violated the "Code of Ethics and Professional Conduct" of ACM, the organisation that hands out these awards: computing professionals should "credit the creators of ideas, inventions, work, and artifacts, and respect copyrights, patents, trade secrets, license agreements, and other methods of protecting authors' works." The awardees didn't; instead they credited each other (and collected citations) for inventions of other researchers. However, ACM "retains the right to revoke an Honor previously granted if ACM determines that it is in the best interests of the field to do so." So I guess they should be stripped of their awards.
Jazzyear: Do you think they directly stole your ideas?
Schmidhuber: It is well-known that plagiarism can be “intentional and reckless” or “unintentional.” However, science has a well-established way of dealing with "multiple discovery" and plagiarism, be it unintentional or not, based on facts such as time stamps of publications and patents. And if you unintentionally reinvent something previously published, you must publish a corrigendum and credit the original inventor in all future papers and presentations. Failing to do so disqualifies you as a scientist. In a mature field such as math, you'd never get away with plagiarism. However, the field of machine learning seems still rather immature in comparison. Anyway, science is self-correcting, and we'll see that in machine learning, too. Sometimes it may take a while to settle disputes, but in the end, the facts must always win.
Schmidhuber and the Deep Learning Trio
Jazzyear: Criticizing prominent figures could lead to negative comments about you online. Do you read these comments? Do you Google yourself?
Schmidhuber: Richard Feynman, the famous physicist, once wrote a book: "What Do You Care What Other People Think?" The only thing that counts in science are the facts. If the facts are known, why should you care for misleading anonymous comments by trolls on the web?
Some people resort to personal attacks when they can’t refute fact-based information, following the adage, “If you can’t argue with the facts, attack the messenger.” Fortunately, unlike politics, science is immune to personal attacks. Science isn’t democratic. If 100 people claim one thing and one person presents the opposite with factual support, the latter wins.
The famous singer Elvis Presley said, “Truth is like the sun. You can shut it out for a time, but it ain’t goin’ away.
Jazzyear: Are you jealous of the American scientists? They might be richer than you.
Schmidhuber: Jealous? Richer? How on earth did you come up with this idea? Also, wealth means nothing in science. Einstein, the most famous scientist of all time, wasn’t rich. Still, they named him “person of the century.” No, I am just happy that the Americans and many others are using our methods so much.
4. Self-replicating, self-improving machine civilizations
Jazzyear: Do you have any mentors or role models in the scientific community?
Schmidhuber: In the 1970s, as a teenager, I initially aspired to be a physicist like my awesome idol, Einstein. A few years later, I realized that within my lifetime, I might be able to create an AI scientist far more intelligent than any human, capable of solving many problems I couldn’t, thus vastly amplifying my limited creativity. This realization set the course of my life.
Overall, I am a very self-driven person, learning hard lessons from experience. My best mentors have been those who allowed me to pursue my dreams without too much interference.
Jazzyear: Are there any entrepreneurs you like?
Schmidhuber: Sure. I like Elon Musk, who kindly invited me to his wonderful family reunion, and Jensen Huang, whose awesome NVIDIA GPUs my team used in 2010 to make deep learning fast enough to break benchmark records. I also like several other amazing but perhaps less famous entrepreneurs.
Schmidhuber met with Jensen Huang (left)
Jazzyear: In previous reports from the New York Times, it’s been said that your misfortune might be being too early—publishing results years ahead of the powerful and affordable computers we now have. Do you consider your experiences unfortunate?
Schmidhuber: Not at all---had I done it later, someone else might have scooped me! Being ahead of time is great, especially in AI where compute is getting 10 times cheaper every 5 years, so a mere human lifetime is sufficient to see how it all pans out.
Jazzyear: What kind of AGI world do you think children born after 2020 will face?
Schmidhuber: It will be awesome! Our AI is already helping to make human lives longer and healthier and easier, and this trend will accelerate. What's next? It’s true AGI in the physical world, not just today’s AI behind the screen. The physical challenges of the real world are far more complex than those of the virtual one. AI still has a long way to go before it can replace skilled trades like plumbers or electricians. However, there is reason to believe AI in the physical world will soon make significant strides.
A next major step will be self-replicating and self-improving societies of physical robots and other machines. We already have 3D printers that can print copies of parts of themselves. But no 3D printer can make a complete copy of itself, like a living being. To assemble a complete 3D printer, you need many other machines, for example, to take the raw material out of the ground, to refine it, to make the machines that make the machines that help make many unprintable parts of the 3D printer, to screw those parts together, and so on. Most importantly, you still need lots of people to oversee and manage all this, and to fix broken machines.
Eventually, however, there will be entire societies of clever and not-so-clever physical machines that can collectively build from scratch all the things needed to make copies of themselves, mine the raw materials they need, repair broken robots and robot factories, and so on. Basically, a machine civilisation that can make copies of itself and then, of course, improve itself. Basically, I am talking about a new form of life, about self-replicating, self-maintaining, self-improving hardware, as opposed to the already existing, self-improving, machine-learning software.
There will be enormous commercial pressure to create such life-like hardware, because it represents the ultimate form of scaling, and its owners will become very rich, because economic growth is all about scaling.
Of course, such life-like hardware won't be confined to our little biosphere. No, variants of it will soon exist on other planets, or between planets, e.g. in the asteroid belt. As I have said many times in recent decades, space is hostile to humans but friendly to suitably designed robots, and it offers many more resources than our thin layer of biosphere, which receives less than a billionth of the energy of the Sun. Through life-like, self-replicating, self-maintaining hardware, the economy of our solar system will become billions of times larger than the current tiny economy of our biosphere. And of course, the coming expansion of the AI sphere won’t be limited to our tiny solar system.
Jazzyear: What are the most pressing issues in AI safety and ethics today? Can AI pose threats comparable to nuclear weapons?
Schmidhuber: AI can indeed be weaponized, as recent conflicts involving cheap AI-driven drones have confirmed. But currently, AI does not pose new existential threats. As I have said for decades: we should be much more afraid of 60-years old technology in form of hydrogen bombs mounted on rockets, which can wipe out a big city within seconds, without any AI.
Jazzyear: Some believe we have no room for trial and error with AGI. What can we do now to ensure safety?
Schmidhuber: This is like saying: let’s not have any more children because we cannot afford trial and error; we cannot simply create yet another human baby that might become a serial killer or develop extremely dangerous AI… By educating babies and AIs in a friendly, rational, and responsible manner, we greatly increase their chances of benefiting society rather than harming it.
Jazzyear: In 2024, what new judgments do you have about AGI?
Schmidhuber: Since the 1970s, I’ve been saying that AI will soon become much smarter than humans, and that almost all AIs will soon be far from Earth where most of the physical resources are that they need to create even more and larger AIs. Self-driven AIs will first take over the solar system, and then the galaxy, and then within a few tens of billions of years the entire visible universe, in a way where humans cannot follow. I’ve been saying this for decades, since my teenager times. The only difference is that more people are listening now. In the 1970s, my mom used to say I am crazy when I explained that to her. In the 1980s, my fellow students said I am crazy. But recently, many have stopped calling me crazy because suddenly they think AGI is very close.
Jazzyear: Can you offer any advice to Chinese startups or young Chinese scientists?
Schmidhuber: Sure! Study our old and recent papers and my AI Blog.
*Miss Jia, founder and CEO of Jazzyear, also contributed to the story.
-
63535
-
8
-
10
-
0